Role of Data Analytics in Optimizing Your Compensation Process

Data analytics plays a crucial role in optimizing the compensation process of an organization. By analyzing compensation data, organizations can identify trends, evaluate the effectiveness of their compensation programs, and make data-driven decisions about their compensation strategy. This allows organizations to remain competitive in attracting and retaining top talent while ensuring fair employee compensation.
Additionally, data analytics can help identify pay gaps, and address equity issues, contributing to a more diverse and inclusive workforce. By tracking metrics such as employee retention rates, engagement levels, and productivity, organizations can also measure the effectiveness of their compensation programs and adjust their strategy accordingly.
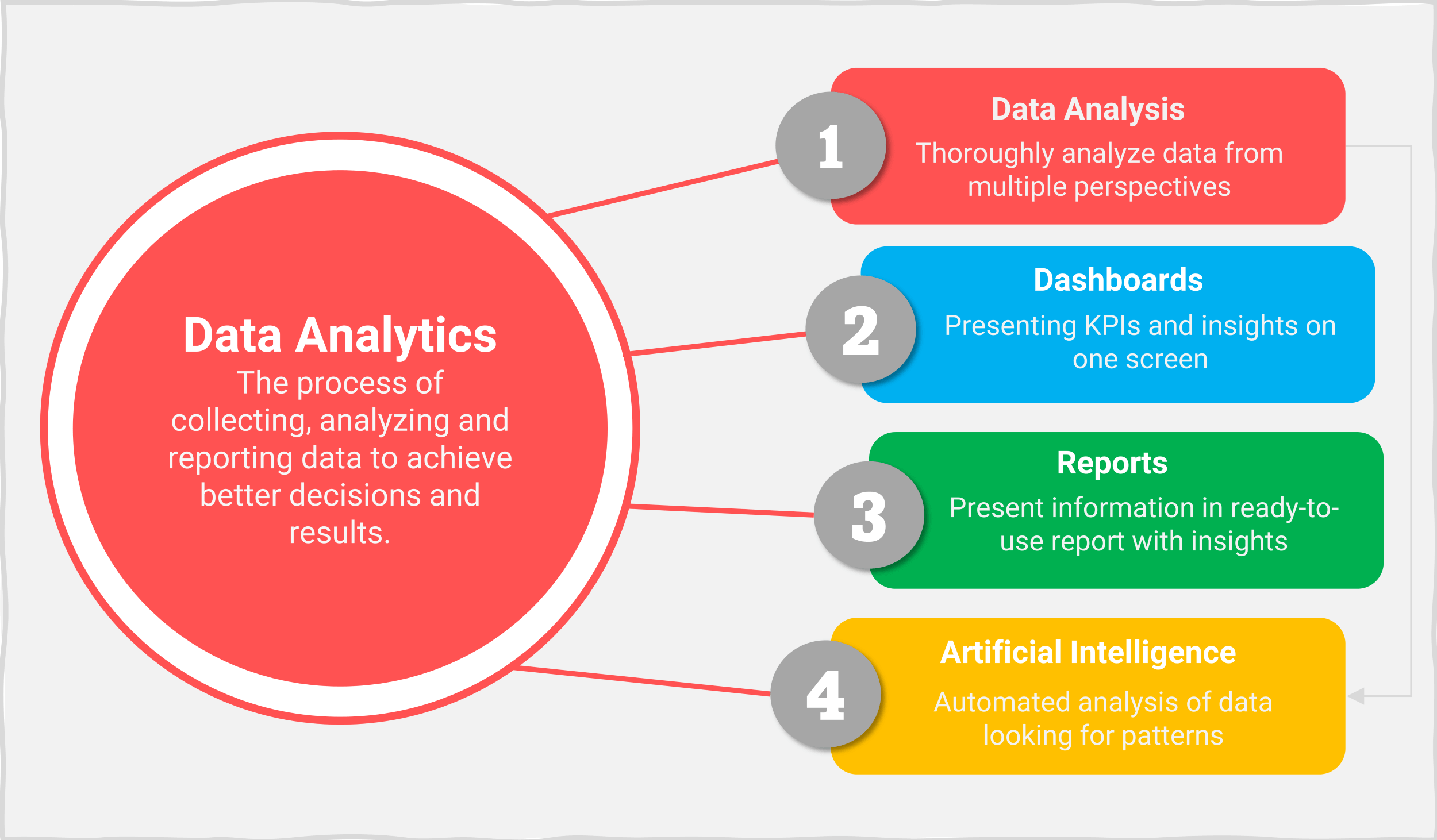
Importance Of Data Analytics In Compensation Process Optimization
Data analytics is critical in optimizing the compensation process of an organization for several reasons:
- Fact-based decision-making: Data analytics provides accurate and reliable information to help organizations make informed decisions about their compensation strategy. By analyzing data, organizations can identify compensation trends, evaluate the effectiveness of their compensation programs, and make data-driven decisions about their compensation strategy.
- Identify pay gaps: Data analytics can help organizations identify pay gaps between employees, which is crucial for addressing pay equity issues. Organizations can analyze compensation data to ensure that all employees are fairly compensated based on their job responsibilities and experience.
- Retain top talent: Data analytics can help organizations identify factors contributing to employee turnover. By analyzing data such as employee satisfaction surveys and performance metrics, organizations can take proactive steps to retain top talent by adjusting their compensation strategy and addressing other factors contributing to employee turnover.
- Remain competitive: By analyzing compensation data, organizations can stay up-to-date with compensation trends in their industry and ensure that their compensation strategy remains competitive. This is essential for attracting top talent and retaining high-performing employees.
- Evaluate the ROI of compensation programs: Data analytics can help organizations evaluate their compensation programs' return on investment (ROI). Organizations can determine which compensation programs are worth the investment by analyzing compensation data and tracking metrics such as employee retention rates and productivity levels.
In summary, data analytics is essential for optimizing the compensation process of an organization. By providing accurate and reliable information, data analytics can help organizations make informed decisions about their compensation strategy, identify pay gaps, retain top talent, remain competitive, and evaluate the ROI of their compensation programs.
Overview Of The Compensation Process

The compensation process refers to determining and administering employee compensation and benefits. It includes the following key steps:
- Job analysis and evaluation: This involves analyzing the duties, responsibilities, and requirements of each job within the organization and evaluating them to determine their relative worth.
- Setting compensation strategy: Based on the job analysis and evaluation results, the organization will determine its compensation strategy, which includes the types of compensation and benefits it will offer and the level of compensation for each position.
- Determining individual compensation: Based on the compensation strategy, the organization will determine the compensation for each employee, taking into account factors such as performance, experience, and market data.
- Administering compensation: Once compensation has been determined, it must be administered through payroll processes and benefits administration.
- Monitoring and adjusting: The compensation process is not a one-time event. It requires ongoing monitoring and adjustment to remain effective and competitive in attracting and retaining top talent.
The compensation process is critical to an organization's ability to attract and retain top talent and ensure that employees are fairly compensated. By following a structured and well-designed compensation process, organizations can ensure they are competitive in the market and maintain a motivated and engaged workforce.
What Are Some Fundamental Challenges in Compensation Process?
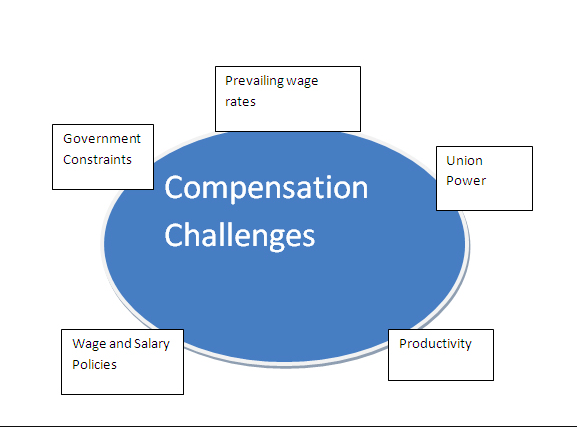
The compensation process involves determining the monetary and non-monetary rewards an organization provides its employees in exchange for their work. The strategy aims to attract, motivate, and retain a talented workforce. This report identifies and explains five key challenges faced in the compensation process.
Market competitiveness
Challenge: Ensuring compensation packages are competitive within the industry and the specific labor market.
Explanation: Organizations must research and analyze competitors' compensation packages to attract and retain top talent. This requires continuous benchmarking and staying up-to-date with market trends and salary surveys.
Internal equity
Challenge: Establishing fair and consistent compensation structures within the organization.
Explanation: Organizations must ensure that employees performing similar roles with comparable levels of responsibility and experience receive equitable pay. This involves creating a comprehensive job evaluation system and developing salary scales based on job roles, performance, and experience.
Legal and regulatory compliance
Challenge: Adhering to labor laws and regulations related to compensation.
Explanation: Organizations must comply with all relevant laws and regulations, such as minimum wage, overtime pay, and equal pay for equal work. Failure to comply can result in financial penalties, legal action, and damage to the organization's reputation.
Balancing Cost and Value
Challenge: Develop compensation packages that balance the organization's financial constraints with attracting and retaining talented employees.
Explanation: Organizations must allocate resources efficiently to offer competitive compensation packages while controlling labor costs. This may involve the strategic use of variable pay, benefits, and other non-monetary rewards to enhance the overall value of the compensation package without significantly increasing costs.
Performance-based compensation
Challenge: Designing and implementing effective performance-based compensation systems.
Explanation: Performance-based compensation systems aim to reward employees based on their performance, which can improve motivation and productivity. However, organizations must carefully design these systems to ensure they are fair, transparent, and accurately measure employee performance.
Effective compensation management is critical for attracting, motivating, and retaining a talented workforce. Organizations must address challenges related to market competitiveness, internal equity, legal and regulatory compliance, balancing cost and value, and performance-based compensation to create a successful compensation strategy. Organizations can optimize their human capital investment by addressing these challenges and driving overall business success.
The Role And Benefits Of Data Analytics In Compensation Process Optimization
Data analytics examines, cleans, transforms, and models data to extract useful information, draw conclusions, and support decision-making. In the context of compensation process optimization, data analytics can be crucial in helping organizations make data-driven decisions related to employee compensation. This report outlines the Role and benefits of data analytics in compensation process optimization.
Role of Data Analytics in Compensation Process Optimization

Market analysis and benchmarking: Data analytics can help organizations gather and analyze relevant market data to benchmark their compensation packages against industry standards and competitors. This ensures that the organization remains competitive in attracting and retaining top talent.
Internal equity analysis: By examining internal data, analytics can help identify pay disparities within the organization, ensuring that employees are paid somewhat based on their job roles, experience, and performance. This fosters a sense of fairness and reduces the likelihood of employee dissatisfaction.
Performance measurement: Data analytics can be used to develop and refine performance metrics, which is critical for designing and implementing effective performance-based compensation systems. This allows organizations to reward employees based on performance, driving motivation and productivity.
Legal and regulatory compliance: Data analytics can help organizations monitor and ensure compliance with labor laws and regulations related to compensation. This reduces the risk of non-compliance penalties and legal action.
Cost optimization: By analyzing compensation-related data, organizations can identify areas for cost savings and allocate resources more efficiently. This can help balance the organization's financial constraints by offering competitive compensation packages.
Benefits of Data Analytics in Compensation Process Optimization
Improved decision-making: Data analytics enables organizations to make informed, data-driven decisions about their compensation strategies, helping them allocate resources more effectively and optimize their investment in human capital.
Enhanced employee satisfaction: By using data analytics to ensure internal equity and develop performance-based compensation systems, organizations can foster a sense of fairness and motivate employees, increasing job satisfaction and reducing turnover.
Greater competitiveness: Data-driven compensation strategies enable organizations to stay competitive in the labor market by offering attractive and well-structured compensation packages.
Regulatory compliance: Data analytics helps organizations maintain compliance with labor laws and regulations, reducing the risk of legal issues and financial penalties.
Cost savings: Through data analysis, organizations can identify areas for cost savings and make strategic adjustments to their compensation packages, helping them balance costs while offering attractive rewards to employees.
Data analytics is essential for organizations looking to optimize their compensation processes. By leveraging data-driven insights, organizations can ensure their compensation strategies are competitive, equitable, and cost-effective. Furthermore, data analytics enables organizations to make informed decisions, maintain compliance with labor regulations, and improve employee satisfaction, ultimately contributing to the organization's overall success.
Examples Of How Data Analytics Can Improve The Compensation Process

Salary benchmarking: Data analytics can gather and analyze salary data from various sources, such as industry reports, salary surveys, and competitor information. This enables organizations to benchmark their compensation packages against industry standards and competitors, ensuring they remain competitive in attracting and retaining top talent.
Identifying pay disparities: By analyzing internal compensation data, organizations can identify pay distinctions based on factors such as gender, ethnicity, or department. This helps organizations address these disparities, ensuring employees are paid fairly and promoting a more inclusive workplace.
Evaluating job roles and pay grades: Data analytics can help organizations assess the relative value of different job roles and establish appropriate pay grades. This ensures that employees performing similar functions with comparable levels of responsibility and experience receive equitable pay, fostering a sense of fairness and reducing the likelihood of employee dissatisfaction.
Optimizing benefits packages: By analyzing data on employee preferences, utilization, and costs of various benefits, organizations can design and tailor benefits packages that are cost-effective and valued by employees. This may include adjusting health insurance plans, offering flexible work arrangements, or providing unique perks that cater to the workforce's specific needs.
Performance-based compensation: Data analytics can help organizations develop and refine performance metrics and analyze employee performance data to inform performance-based compensation systems. This enables organizations to reward employees based on performance, driving motivation and productivity.
Employee retention analysis: By analyzing employee turnover data and identifying factors that contribute to attrition, organizations can adjust their compensation strategies to retain talent better. For example, they might introduce retention bonuses, offer more competitive salary increases, or improve career development opportunities.
Workforce planning and budgeting: Data analytics can support workforce planning and budgeting by projecting future compensation costs based on anticipated headcount changes, inflation, and market trends. This helps organizations allocate resources more effectively and optimize their investment in human capital.
Assessing the effectiveness of incentive programs: Data analytics can be used to evaluate the success of incentive programs, such as bonuses or stock options, by measuring their impact on employee performance, engagement, and retention. This allows organizations to make data-driven decisions on adjusting or continuing such programs.
Geographic pay differentials: Data analytics can help organizations determine appropriate compensation adjustments based on the cost of living and other factors in different geographic locations. This ensures that employees are compensated somewhat based on the local labor market and living costs.
Analyzing the impact of compensation changes: Data analytics can be used to assess the effects of changes in compensation strategies, such as introducing a new pay structure or benefit. By monitoring key performance indicators, such as employee engagement, productivity, and turnover, organizations can determine the success of their compensation adjustments and make further refinements if necessary.
Steps In Implementing Data Analytics In Compensation Process Optimization
- Define objectives and goals: Clearly outline the specific objectives and goals for implementing data analytics in compensation process optimization. These goals include improving internal equity, ensuring market competitiveness, or optimizing the cost of the compensation package.
- Assemble a cross-functional team: Form a team of professionals with diverse expertise, including HR, finance, data analytics, and IT. This team will collaborate in designing, implementing, and monitoring the data-driven compensation strategy.
- Collect and consolidate data: Gather compensation-related data from internal and external sources, such as salary surveys, industry reports, competitor information, and internal payroll and performance records. Ensure the data is accurate, up-to-date, and relevant to the organization's goals.
- Clean and preprocess data: Data often needs to be cleaned and preprocessed before analysis. This may involve removing duplicate records, filling in missing data, or transforming data into a suitable format for research.
- Select appropriate analytical tools and techniques: Choose the proper data analytics tools and techniques that best suit the organization's needs and objectives. This may involve selecting statistical methods, machine learning algorithms, or visualization tools
- Analyze the data: Perform the analysis using the selected tools and techniques. This may involve identifying patterns, trends, and correlations related to the organization's compensation goals.
- Interpret and communicate results: Translate the results of the data analysis into actionable insights that inform decision-making. Clearly and concisely communicate these insights to relevant stakeholders, including HR and senior management.
- Implement data-driven compensation strategies: Based on the insights gained from the data analysis, design and implement data-driven compensation strategies that address the organization's goals and objectives. This may involve adjusting salary structures, refining performance metrics, or introducing new benefits.
- Monitor and evaluate outcomes: Regularly monitor the outcomes of the implemented compensation strategies to assess their effectiveness. This may involve tracking key performance indicators (KPIs), such as employee engagement, productivity, and turnover, and comparing them against pre-defined benchmarks.
- Refine and iterate: Continuously refine and adjust the data-driven compensation strategies based on the ongoing monitoring and evaluation of their outcomes. This iterative process helps organizations stay agile and responsive to changes in the market, ensuring their compensation strategies remain effective and competitive.
By following these steps, organizations can successfully implement data analytics in their compensation process optimization, leading to improved decision-making, greater competitiveness, and enhanced employee satisfaction.
Tools And Technologies For Data Analytics In Compensation Process Optimization

Effective data analytics in compensation process optimization requires appropriate tools and technologies. This report presents an overview of various tools and technologies that can facilitate data analytics in the compensation process, grouped into four main categories: data collection and storage, data cleaning and preprocessing, data analysis, and data visualization and reporting.
Data Collection and Storage
Human Resource Information Systems (HRIS): HRIS platforms, such as Workday, BambooHR, and ADP, help organizations store and manage employee data, including compensation and performance records.
Surveys and Benchmarking Tools: Tools like PayScale, Glassdoor, and Salary.com provide access to industry salary data and allow organizations to conduct custom salary surveys.
Databases: Structured database systems, such as SQL databases or NoSQL databases, like MongoDB, can be used to store, manage, and retrieve compensation-related data.
Data Cleaning and Preprocessing
Excel: Microsoft Excel is a widely used spreadsheet tool that offers basic data cleaning and preprocessing functions.
OpenRefine: OpenRefine is an open-source tool that helps easily clean and transform large datasets.
Python or R: Python and R programming languages offer powerful libraries, such as pandas and dplyr, for cleaning and preprocessing data.
Data Analysis
Statistical analysis tools: Software like IBM SPSS, SAS, or R can perform various statistical analyses on compensation data.
Machine Learning Libraries: Python and R offer machine learning libraries, such as sci-kit-learn and caret, which can be used for predictive modeling and clustering in compensation analysis.
Regression analysis: Tools like Stata or R can be employed to analyze regression, helping organizations identify the factors that impact employee compensation.
Data Visualization and Reporting
Tableau: Tableau is a popular data visualization tool that allows users to create interactive and shareable dashboards to communicate insights from compensation data analysis.
Power BI: Microsoft Power BI is a business intelligence platform that helps users create visualizations and reports from multiple data sources.
Datawrapper: Datawrapper is a web-based tool that enables users to create customizable and responsive charts and graphs for presenting compensation data.
The right tools and technologies are crucial for implementing data analytics in compensation process optimization. Organizations should consider factors such as the size and complexity of their compensation data, the required analytical methods, and the desired level of automation and customization when selecting tools. Organizations can enhance their compensation process by utilizing the appropriate tools and technologies and making data-driven decisions that improve competitiveness, fairness, and cost-effectiveness.
Best Practices And Tips For Effectively Using Data Analytics In Compensation Process Optimization
Effectively using data analytics in compensation process optimization requires the implementation of best practices and strategies that maximize the potential of data-driven decision-making. The following section outlines best practices and tips for organizations seeking to optimize their compensation processes using data analytics.
Best Practices and Tips
- Clearly define objectives and goals: Establish specific, measurable, achievable, relevant, and time-bound (SMART) objectives and goals for your data analytics efforts in compensation process optimization. This will help guide your data analysis and ensure your actions are aligned with your organization's overall strategy.
- Prioritize data quality: Ensure the data you collect and analyze is accurate, complete, consistent, and up-to-date. Precise only or exclusive data can lead to good insights and decisions. Establish data governance policies and procedures to maintain data quality.
- Collaborate with stakeholders: Involve key stakeholders, such as HR, finance, and management, in the data analytics process. Collaboration ensures that diverse perspectives are considered, and the insights derived from data analysis align with the organization's objectives and strategies.
- Use multiple data sources: Supplement your internal data with external data sources, such as industry benchmarks, salary surveys, and competitor information. This helps provide a more comprehensive view of the compensation landscape and enhances the accuracy of your insights.
- Employ a mix of analytical techniques: Use a combination of descriptive, predictive, and prescriptive analytics to gain a deeper understanding of the factors affecting your compensation processes. This approach enables you to uncover trends and patterns, make forecasts, and develop actionable recommendations.
- Adopt an iterative approach: Continuously monitor and evaluate the outcomes of your data-driven compensation strategies. Refine and adjust your system based on these evaluations, ensuring your compensation processes remain effective and competitive in changing market conditions.
- Communicate insights effectively: Present your findings and recommendations to stakeholders clearly, concisely, and visually appealingly. Use data visualization tools and techniques to help convey complex information and ensure insights are easily understood and actionable.
- Invest in employee training and development: Equip your HR professionals and other team members with the necessary skills and knowledge to use data analytics tools and techniques effectively. This may involve providing training on statistical methods, data visualization, or programming languages, such as R or Python.
- Ensure data privacy and security: Implement strong data privacy and security policies and practices to protect sensitive employee information. Ensure compliance with relevant data protection regulations, such as the General Data Protection Regulation (GDPR) or the California Consumer Privacy Act (CCPA).
- Stay updated on industry trends: Regularly review industry trends, emerging technologies, and best practices related to data analytics in compensation optimization. This will help you stay ahead of the curve and ensure your organization's compensation strategies remain competitive and current.
Avoiding Common Pitfalls In Data Analytics In Compensation Process Optimization
- Data Quality: Ensure that your data is accurate, complete, and up-to-date. Data quality can lead to correct analysis and misguided decision-making. Establish a data validation process and data governance structure to maintain data quality.
- Data Integration: Integrate data from various sources, such as HRIS, payroll, performance management, and market data, to gain a comprehensive view of the compensation process. Develop a consistent data structure and naming conventions for seamless integration.
- Clear Objectives: Define clear objectives for the compensation process optimization project. This will help you focus on the correct data and analytics techniques, avoiding unnecessary analysis or scope creep
- Appropriate Metrics: Select metrics relevant to your objectives and widely accepted within your industry. Using the right or non-standard metrics can lead to accurate results and better decisions.
- Use advanced analytics techniques, such as regression analysis, machine learning, and predictive modeling, to identify trends, correlations, and patterns in your data. This will enable you to make more informed decisions and optimize the compensation process.
- Data Privacy and Security: Ensure data privacy by anonymizing sensitive information and implementing strict access controls. This will protect employee confidentiality and help you comply with data protection regulations
- Understand Limitations: Be aware of the limitations of your data and analytics techniques. This will help you interpret the results accurately and make more informed decisions. Always question your assumptions and validate your findings through multiple data sources or methods.
- Collaboration: Involve stakeholders from HR, finance, and other relevant departments in the compensation process optimization project. This will ensure everyone is on the same page and help avoid misunderstandings or miscommunications.
- Change Management: Plan for change management when implementing the results of your data analytics. This includes providing training, setting expectations, and monitoring the impact of the changes on the organization.
- Continuous Improvement: Monitor the performance of the optimized compensation process regularly and adjust it as needed. This will ensure the process remains practical and relevant in changing business needs and market condition
Conclusion
In conclusion, data analytics is pivotal in optimizing your compensation process, enabling organizations to make informed decisions that drive employee satisfaction, retention, and performance. By harnessing the power of data, organizations can gain valuable insights into compensation trends, identify areas for improvement, and develop targeted strategies to address these challenges. A well-optimized compensation process is vital for remaining competitive in the market and attracting top talent.
However, it is essential to recognize that compensation optimization is not a one-time exercise. Ongoing evaluation and improvement of the compensation process using data analytics is crucial to maintaining its effectiveness in a constantly changing business environment. Regularly monitoring and adjusting the compensation process helps ensure it remains responsive to market shifts, organizational goals, and employee needs. Embracing a data-driven approach to compensation management enables organizations to stay agile, make better decisions, and foster a more engaged and productive workforce.
Find out how Compport can help you manage all your Compensation Management process, book a demo today!
%2520(6)%2520(2).png)
Frequently Asked Questions
Q1. What is the role of data analytics in optimizing the compensation process?
Data analytics plays a crucial role in optimizing the compensation process of an organization. By analyzing compensation data, organizations can identify trends, evaluate the effectiveness of their compensation programs, and make data-driven decisions about their compensation strategy.
Q2. How can data analytics help identify pay gaps and address equity issues?
Data analytics can help organizations identify pay gaps between employees, which is crucial for addressing pay equity issues. Organizations can analyze compensation data to ensure that all employees are fairly compensated based on their job responsibilities and experience.
Q3. How does data analytics contribute to retaining top talent?
Data analytics can help organizations identify factors contributing to employee turnover. By analyzing data such as employee satisfaction surveys and performance metrics, organizations can take proactive steps to retain top talent by adjusting their compensation strategy and addressing other factors contributing to employee turnover.
Q4. How can data analytics optimize benefits packages in the compensation process?
By analyzing data on employee preferences, utilization, and costs of various benefits, organizations can design and tailor benefits packages that are cost-effective and valued by employees. This may include adjusting health insurance plans, offering flexible work arrangements, or providing unique perks.
Q5. What are the steps involved in implementing data analytics in the compensation process optimization?
The steps involved in implementing data analytics in the compensation process optimization include defining objectives and goals, assembling a cross-functional team, collecting and consolidating data, cleaning and preprocessing data, selecting appropriate analytical tools and techniques, analyzing the data, interpreting and communicating results, implementing data-driven compensation strategies, monitoring and evaluating outcomes, and refining and iterating the strategies as necessary.
Recommended articles
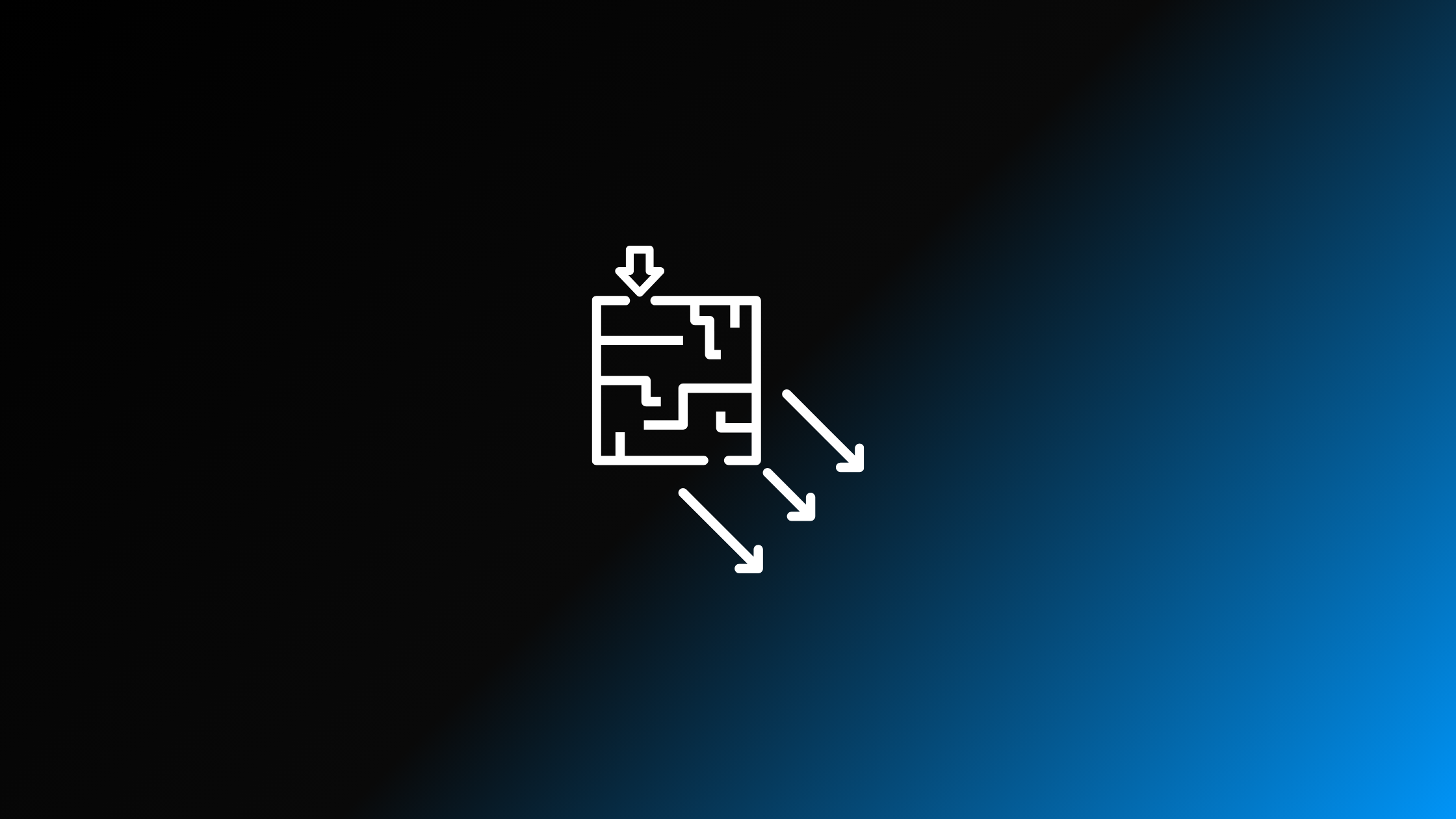
8 Best Practices to Unravel the Complexity of Management Compensation Structures
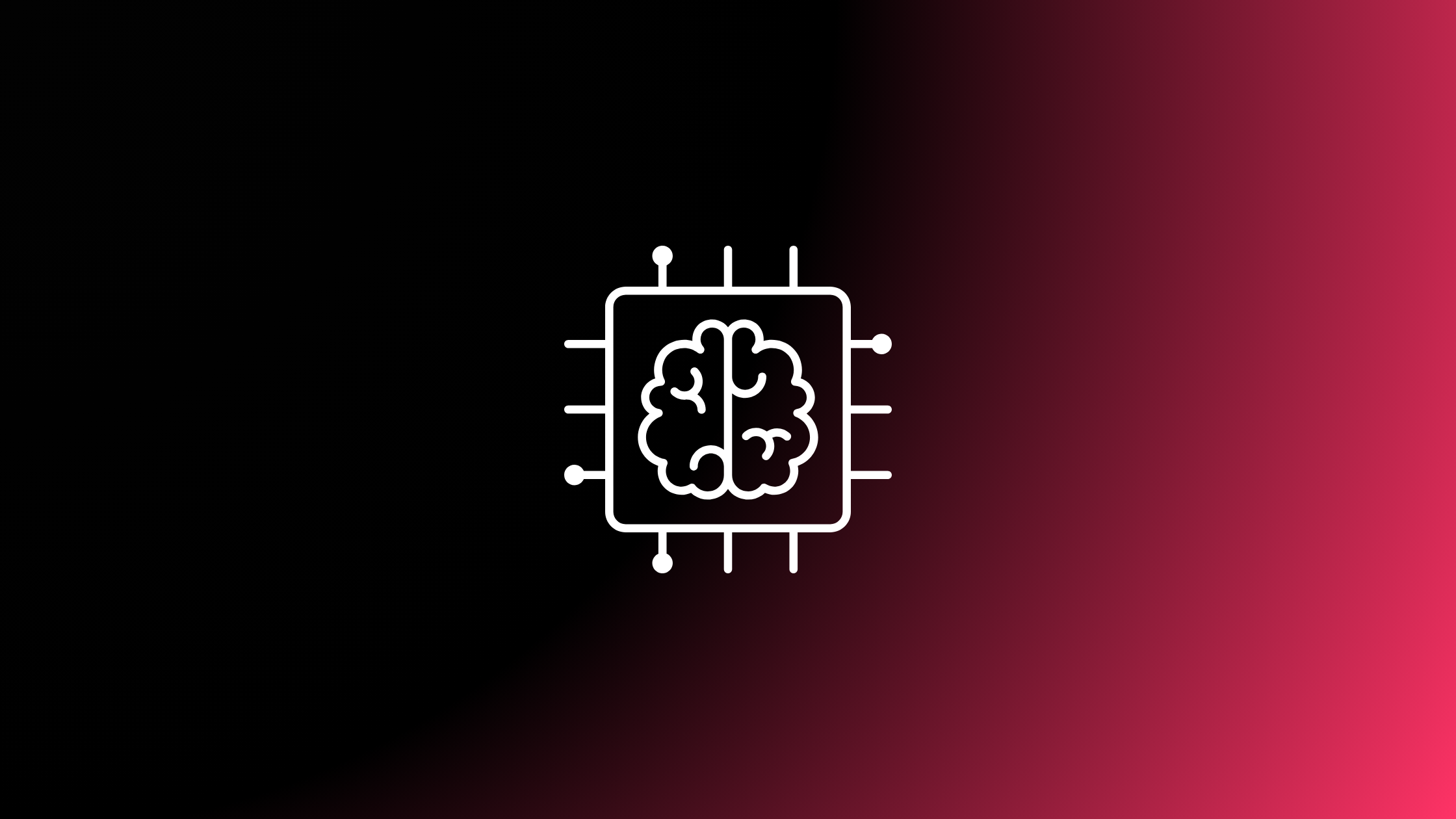
When AI Meets Compensation: Unveiling the Limitations of Generative AI in Compensation Management
