When AI Meets Compensation: Unveiling the Limitations of Generative AI in Compensation Management
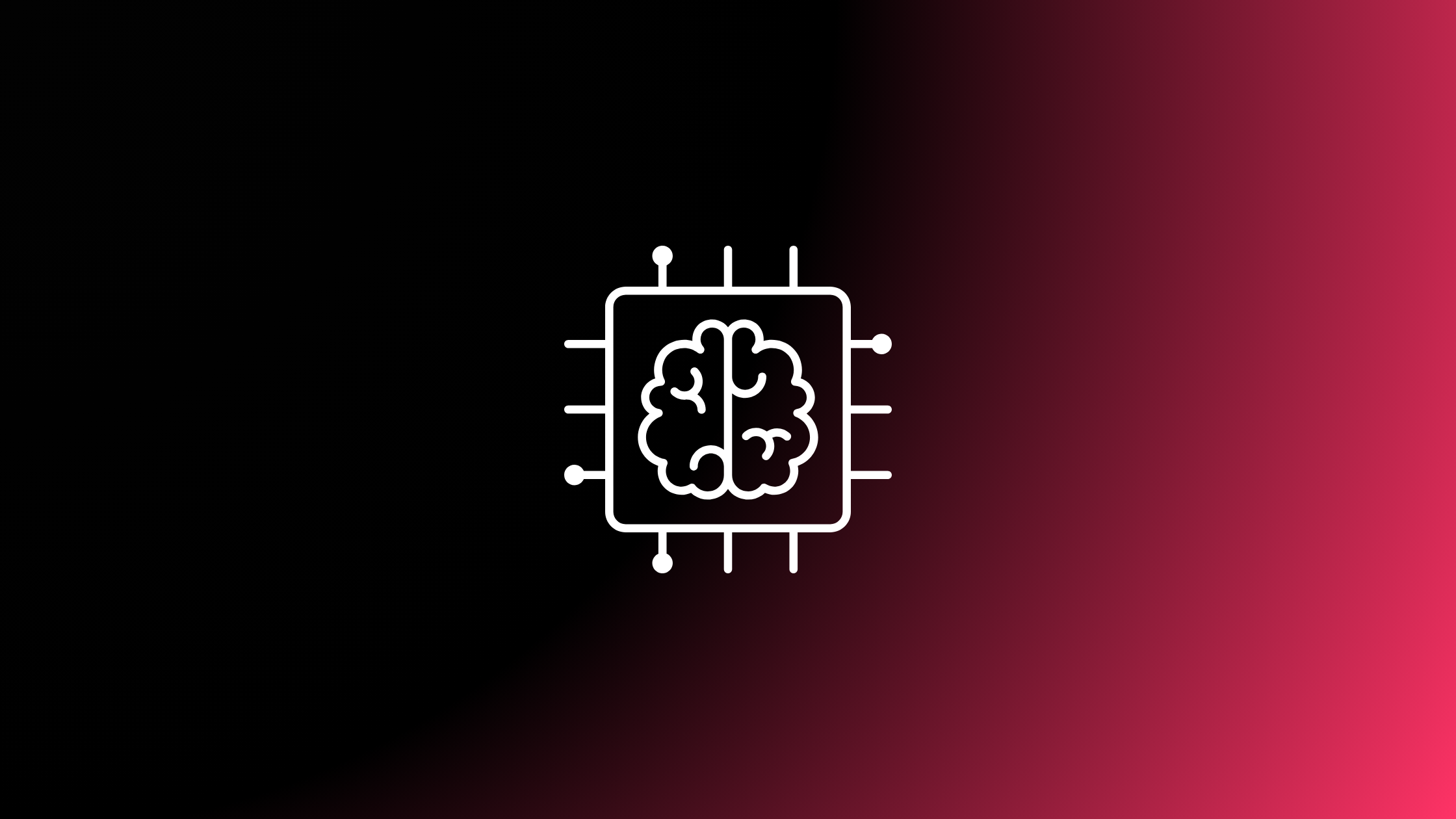
In today's rapidly evolving business landscape, the integration of artificial intelligence (AI) has become increasingly prevalent across various industries, including compensation management. The promise of AI in automating processes, analyzing vast amounts of data, and making data-driven decisions has captured the attention of organizations seeking to optimize their compensation practices. However, it is essential to understand that while generative AI holds significant potential, it also comes with its limitations.
Understanding Generative AI in Compensation Management
Generative AI refers to the use of algorithms that can analyze data patterns, predict outcomes, and automate certain tasks within compensation management. By leveraging generative AI, organizations can gain insights into compensation trends, design equitable pay structures, and streamline compensation processes. The ability to analyze vast amounts of data efficiently and make data-driven decisions is one of the key advantages of generative AI in compensation management.
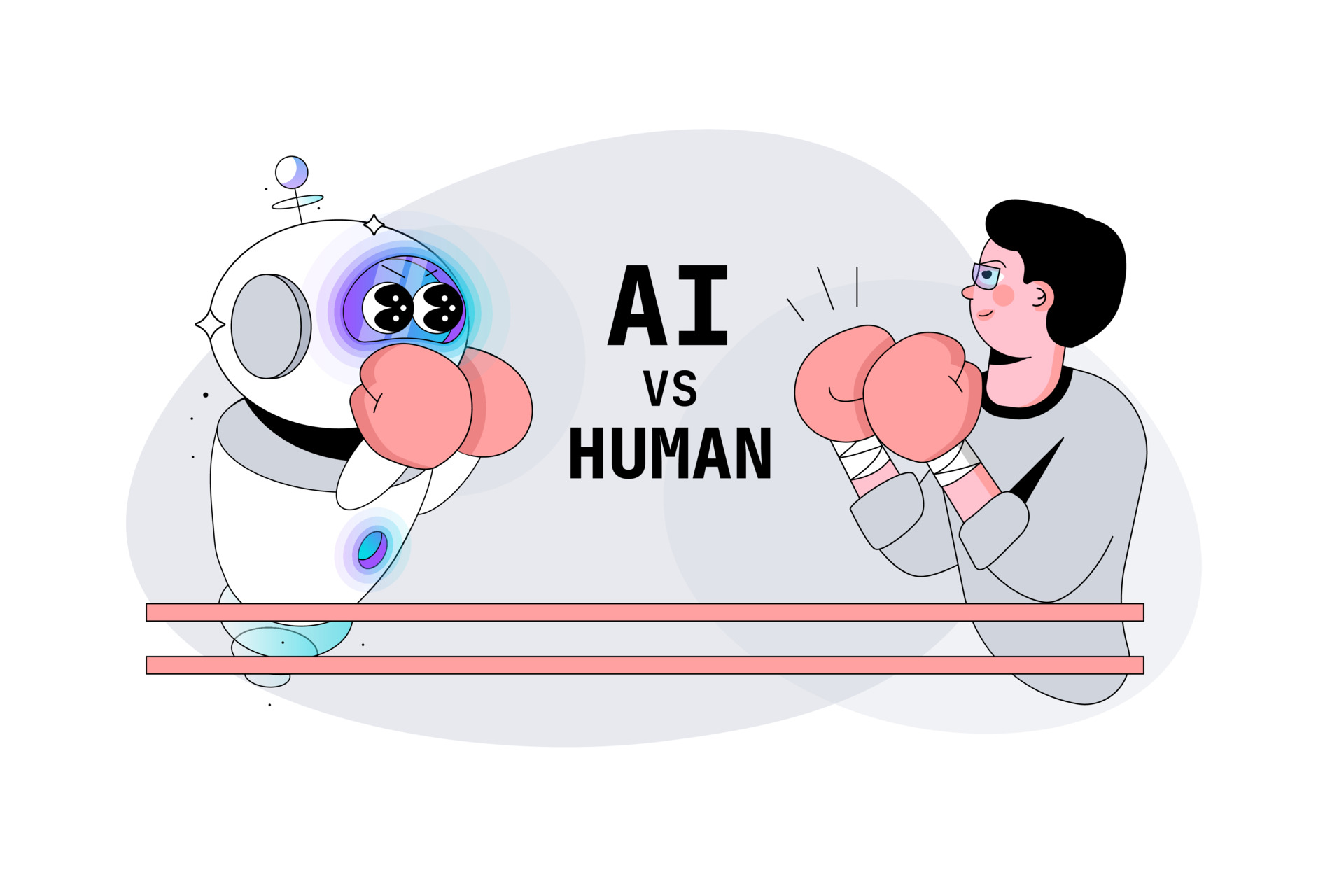
Limitations of Generative AI in Compensation Management
While generative AI brings numerous benefits to compensation management, it is crucial to recognize its limitations. One of the primary limitations is the complexity of compensation decisions. Compensation decisions often involve subjective factors, individual circumstances, and organizational context that may not be easily captured by AI algorithms. Elements such as performance intangibles, employee potential, and cultural fit are challenging to quantify and incorporate accurately into generative AI models.
Here are some key pointers detailing the limitations of using generative AI in compensation management:
Complexity of Compensation Decisions
Compensation decisions involve various factors, such as individual performance, experience, skills, and market conditions. Generative AI models may struggle to capture the nuanced aspects that influence compensation, such as employee potential, cultural fit, and subjective evaluations. The complexity of compensation decisions often requires human judgment and contextual understanding, which may be challenging for AI algorithms to replicate accurately.
Subjectivity and Individual Circumstances
Compensation decisions are not solely based on objective metrics. They also consider subjective factors, such as employee contributions, potential, and future growth prospects. Generative AI models primarily rely on historical data patterns and may not adequately account for individual circumstances or exceptional performance. Human judgment is crucial in assessing unique situations and making fair compensation decisions.
Limited Availability of Data
Generative AI algorithms rely heavily on data availability and quality. In compensation management, certain data points may be limited or incomplete, making it challenging for AI models to derive accurate insights. For example, data on employee satisfaction, work-life balance, or intangible contributions may not be readily available or quantifiable, limiting the effectiveness of generative AI in capturing the full compensation landscape.

Ethical Considerations and Bias
Generative AI models can inadvertently perpetuate biases present in historical data, leading to unfair compensation outcomes. Biases related to gender, race, age, or other protected characteristics can be inadvertently learned by AI algorithms and perpetuated in compensation decisions. It is essential to ensure that generative AI models are designed to be fair, transparent, and free from discrimination, and regular audits should be conducted to identify and mitigate any biases that may arise.
Lack of Contextual Understanding
Generative AI models primarily rely on statistical patterns and correlations within the data. However, they may lack the ability to fully grasp the contextual understanding and intricate dynamics within an organization. Factors such as team dynamics, project complexities, and organizational goals often require human interpretation and judgment to make informed compensation decisions.
Dynamic Nature of Compensation
Compensation management is a dynamic process influenced by various external and internal factors, including market trends, economic conditions, and organizational changes. Generative AI models may struggle to adapt quickly to these dynamic changes, potentially leading to outdated or suboptimal compensation recommendations. Human professionals possess the agility and adaptability to respond to evolving compensation needs more effectively.
The Importance of Human Judgment and Context
While generative AI has the potential to augment compensation decision-making, it is crucial to recognize the continued importance of human judgment and contextual understanding. Human professionals possess expertise, intuition, and nuanced insights that are invaluable in evaluating compensation factors beyond what data alone can provide. The ability to consider individual circumstances, organizational dynamics, and the human element in compensation decisions remains a critical aspect of effective compensation management.
Conclusion
To leverage the benefits of generative AI while addressing its limitations, organizations should strive for a balanced approach that combines human expertise with AI capabilities. Generative AI can be a powerful tool to support compensation decision-making by providing data-driven insights and recommendations. However, it should never replace the role of compensation professionals. Human oversight, continuous monitoring, and fine-tuning of AI models are essential to ensure fairness, accuracy, and alignment with organizational goals.
Frequently Asked Questions
1: What is generative AI in compensation management, and how can it benefit organizations?
Generative AI refers to the use of algorithms to automate tasks, analyze data patterns, and make predictions in compensation management. It can provide insights into compensation trends, facilitate the design of equitable pay structures, and streamline compensation processes, ultimately optimizing compensation practices.
2: What are the limitations of using generative AI in compensation management?
While generative AI offers significant advantages, it is important to understand its limitations. Factors such as the complexity of compensation decisions, subjective and individual circumstances, limited data availability, ethical considerations and bias, lack of contextual understanding, and the dynamic nature of compensation can pose challenges for generative AI models.
3: How does generative AI handle the complexity of compensation decisions?
Compensation decisions involve various factors that may be challenging for generative AI models to capture accurately. Elements such as employee potential, cultural fit, and subjective evaluations require human judgment and contextual understanding, which can be difficult for AI algorithms to replicate.
4: Can generative AI account for subjective and individual circumstances in compensation management?
Generative AI primarily relies on historical data patterns, making it less equipped to account for subjective factors and individual circumstances. Human judgment plays a crucial role in evaluating unique situations and making fair compensation decisions based on factors like employee contributions, potential, and growth prospects.
5: How does the availability of data impact the effectiveness of generative AI in compensation management?
Generative AI algorithms heavily rely on the availability and quality of data. In compensation management, certain data points, such as employee satisfaction or intangible contributions, may be limited or difficult to quantify, which can limit the effectiveness of generative AI in providing comprehensive insights.
6: What measures are in place to address ethical considerations and biases in generative AI-based compensation management?
Generative AI models can unintentionally perpetuate biases present in historical data, leading to unfair compensation outcomes. Regular audits and designing AI models to be fair, transparent, and free from discrimination are essential to identify and mitigate any biases related to gender, race, age, or other protected characteristics.
Find out how Compport can help you manage all your Compensation Management and Rewards Tech needs, book a demo today!
%2520(6)%2520(2).png)